Handbook on Intelligent Healthcare Analytics
Здесь есть возможность читать онлайн «Handbook on Intelligent Healthcare Analytics» — ознакомительный отрывок электронной книги совершенно бесплатно, а после прочтения отрывка купить полную версию. В некоторых случаях можно слушать аудио, скачать через торрент в формате fb2 и присутствует краткое содержание. Жанр: unrecognised, на английском языке. Описание произведения, (предисловие) а так же отзывы посетителей доступны на портале библиотеки ЛибКат.
- Название:Handbook on Intelligent Healthcare Analytics
- Автор:
- Жанр:
- Год:неизвестен
- ISBN:нет данных
- Рейтинг книги:4 / 5. Голосов: 1
-
Избранное:Добавить в избранное
- Отзывы:
-
Ваша оценка:
- 80
- 1
- 2
- 3
- 4
- 5
Handbook on Intelligent Healthcare Analytics: краткое содержание, описание и аннотация
Предлагаем к чтению аннотацию, описание, краткое содержание или предисловие (зависит от того, что написал сам автор книги «Handbook on Intelligent Healthcare Analytics»). Если вы не нашли необходимую информацию о книге — напишите в комментариях, мы постараемся отыскать её.
The book explores the various recent tools and techniques used for deriving knowledge from healthcare data analytics for researchers and practitioners. A Handbook on Intelligent Healthcare Analytics
Handbook on Intelligent Healthcare Analytics — читать онлайн ознакомительный отрывок
Ниже представлен текст книги, разбитый по страницам. Система сохранения места последней прочитанной страницы, позволяет с удобством читать онлайн бесплатно книгу «Handbook on Intelligent Healthcare Analytics», без необходимости каждый раз заново искать на чём Вы остановились. Поставьте закладку, и сможете в любой момент перейти на страницу, на которой закончили чтение.
Интервал:
Закладка:
Keywords:Artificial intelligence, big data, Improved Bayesian Hidden Markov Frameworks (IBHMF), hidden state, knowledge engineering, weather forecasting
2.1 Introduction
Catastrophic damage has been caused by natural hazards along with loss in a socioeconomic way, thereby depicting the increase in trend. Several disasters pose challenges to officials working in the disaster management field. These challenges may include resources unavailability and limited workforce, and these limitations force them from changing the policies toward managing the disasters [1].
The amount of data generated is huge in size including the real along with the simulation data. These data can be used in supporting disaster management. The technological advancement like data generated from social media as well as remote sensing is huge in size and also is real data. In certain times, these real data are scarce and lead us to usage of simulation data. Several computational models can be used in generation of simulation data that can be used in estimation of impact produced due to disaster. It is much necessary to acquire big data, manage it, and process within a short time span for effective management of disaster irrespective of the type of data being used. For this reason, artificial intelligence (AI) methods can be employed for analyzing the huge volume of data for extracting useful information. Such methods have gained popularity while they support the process of making decisions in case of disaster management [5, 6].
The usage of big data for managing disasters is still evolving. The main challenge for a scientist in today’s technological world is handling the huge volume of information that is being generated during disaster time. When the volume of data is being increased, traditional systems ( Figure 2.1) employed for storing and data processing are not able to perform better. The factors affecting their working include scalability along with data availability [8].
The storage systems at the present time are diverse in nature, and when it comes to collaboration, they provide much less scope. This leads to the necessity of methods that can be employed for integration, aggregation, and visualization of data. Also, the decisions taken need to be optimized as their quality is based on the available data quality. It is much necessary to organize data followed by storage and analysis of disaster data for further investigation [11].
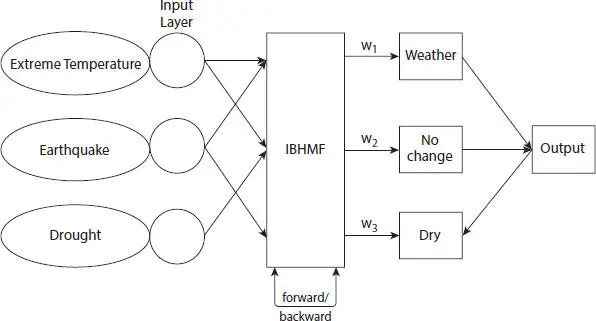
Figure 2.1 Traditional Bayesian Neural Network disaster prediction from the dataset.
2.1.1 Knowledge Engineering in AI and Its Techniques
AI can be useful for disaster management, wherein it can be further classified as the following categories: supervised model, unsupervised model, deep learning, and reinforcement learning along with optimization.
2.1.1.1 Supervised Model
Training is done using the human input on the data that is pre-existing in case of algorithms, representing the supervised model. Such models can represent a function by utilizing the methods such as classification for predicting the output value. This is due to the training data that is labeled and also the input as well as output pairs. The main advantages of these models include extraction of information and recognition of objects, patterns, and speech [17].
2.1.1.2 Unsupervised Model
Statistical methods are being used for extraction of hidden structure from the unlabeled data in unsupervised models. Human input is also absent here, and they are utilized in detection of abnormal data along with reduction of dimension of the data. Its applications include clustering as well problems such as data aggregation. The unlabeled data can be partitioned as several groups depending on the similarity feature. This recognition of patterns can be done using the clustering algorithms. On the other hand, the algorithms employed for reduction of dimension like PCA (Principal Component Analysis) play a significant role in data complexity reduction, which, in turn, helps in avoiding overfitting [18].
2.1.1.3 Deep Learning
Input data can be used for extracting the features by using the multiple layers and such algorithm classes constitute deep learning [20]. The learning performance is improved with a wide scope of application [3, 10]. The main disadvantage of using the algorithms in deep learning is that they take more time for training the data. These algorithms can be employed for solving problems such as assessment of damage, detecting motion, recognizing facial expressions, prediction of transportation, and also supports the management of disaster using natural language processing (NLP). Both Recursive and Recurrent Neural Networks can be used for applying along with NLP. At the same time, Convolutional Neural Networks can be used in image classification, text processing, and computer vision [19].
2.1.1.4 Deep Reinforcement Learning
Problems that are goal-oriented can be solved by taking decisions in the order of a sequence is performed by algorithms in reinforcement learning [11]. Here, learning can be carried out based on the reinforcement series, and modeling is based on decision processes such as Markov. These algorithms play a major role in finding solutions for the problems where the decisions to be made need to be in sequence and their applications extend to management of resources and controlling traffic light. Major problem in such algorithms is the preparation of the environment for training the data and performing tasks based on them. When a deep neural network (NN) is combined with reinforcement learning, it is referred to as deep reinforcement learning. The major goal of such algorithms is creation of software agents who learn themselves for establishing the policies in a successful manner. Additionally, they help in attaining the rewards pending in long term. In terms of performance, deep reinforcement learning solves the problems including sequential tasks that are complex such as robotics and computer vision. The need of more training data along with time for training for attaining better performance is a major drawback of deep reinforcement learning as they are computationally expensive. Data can be also be secured with usage of grids [6, 21, 24].
2.1.1.5 Optimization
Optimization helps in identifying the suitable model using objective function and is considered to be one of the important methods in case of disaster management. Several optimization methods are available, and based on their performance, they can be recommended for further applications [24].
2.1.2 Disaster Management
Disaster management starts with the phase of mitigation where activities are related to prevention of emergencies in the future including the consequences. It is the initial phase in managing the disasters. The activities related to mitigation includes enforcement of standards, providing hospital care along with shelters, and providing education for the public. This awareness can help people and also the stakeholders to deal with hazards and strategies for mitigation. The next phase is the preparedness, where the disaster is going to happen. These include the activities that can help in saving the lives of people along with helping the rescue operations like food stocking, providing emergency data, and evacuations. Following this phase comes the response stage. This stage takes place mainly at the time of disaster: evacuation of areas that are being threatened, fire fighting works, efforts such as search along with rescue, and management of shelter including assistance using humanitarians. When the disaster has occurred, the recovery stage deals with repair and the efforts related to reconstruction for returning the life to a normal level. Actions in case of recovery are cleaning the debris, assessment of damage, and reconstruction of infrastructure. It also includes assistance related to finance from the agencies of government or companies that provide insurance.
Читать дальшеИнтервал:
Закладка:
Похожие книги на «Handbook on Intelligent Healthcare Analytics»
Представляем Вашему вниманию похожие книги на «Handbook on Intelligent Healthcare Analytics» списком для выбора. Мы отобрали схожую по названию и смыслу литературу в надежде предоставить читателям больше вариантов отыскать новые, интересные, ещё непрочитанные произведения.
Обсуждение, отзывы о книге «Handbook on Intelligent Healthcare Analytics» и просто собственные мнения читателей. Оставьте ваши комментарии, напишите, что Вы думаете о произведении, его смысле или главных героях. Укажите что конкретно понравилось, а что нет, и почему Вы так считаете.