Keywords :Computer aided diagnosis, K-nearest neighbor, artificial neural network, electroencephalography, Internet of Things, support vector machine, brain modeling feature exraction
IoT (Internet of Things) is utilized as a part of a great deal of medical uses. A portion of the uses of Internet of Things are savvy stopping, shrewd home, brilliant city, keen condition, mechanical spots, horticulture fields and wellbeing observing procedure [38]. One such application in medicinal services to screen the patient’s wellbeing status by means of Internet of Things makes therapeutic gear more effective by permitting ongoing checking of patient’s wellbeing, in which sensor get information of patient’s and decreases the human blunder. The Internet of Things in the therapeutic field draws out the answer for compelling continuous checking of rationally impaired individual at diminished cost and furthermore lessens the exchange off between tolerant result and infection administration [33]. So far we have seen the wellbeing observing framework which gathers data of fundamental parameters, for example, heartbeat, temperature, circulatory strain and development parameters. The medical data stored in cloud in the form of huge dataset, need to analyze and predict the diseases based on IoT data is very important [1, 37].
The progress of science has driven every individual to mine and consume medical data for analyses of business, customer, bank account, medical, etc. made privacy break or intrusion also in most circumstances. The IoT-based medical data is all over in the pattern of text, number, images and videos [35, 36]. This type of data continues to grow bigger, thereby organizing these data as a necessary process. The collected enormous data should produce logical use unless it would be waste of time, effort and storage. The action of grabbing or collection of huge data is called datafication. Clinical data can be used effectively as it is datafied. The organizing of data alone cannot make useful but should identify what can be performed by its use. Optimal processing power, analytical capabilities and skills are needed for squeezing essential information from medical data. The data mining features are shown in Figure 1.1.
Medical data is of various types, formats and shapes which are brought together from various sources. Data Analytics is the action of studying and extracting big data which can yield functional and business knowledge in a remarkable form. The behavior of business is reconstituted in different ways by big data analytics [15]. Approaches like information technology, statistics, quantitative methods and various methods are used by medical analytics to deliver results. Data mining analytics is divided into three main types. They are descriptive analytics, predictive analytics and prescriptive analytics. The traditional database systems are not sufficient to progress huge data characteristics (elements) [2].
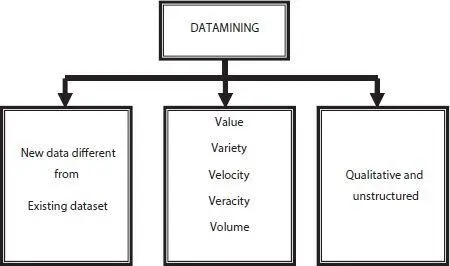
Figure 1.1 Data mining features.
1.1.1 Descriptive Analytics
Descriptive analytical type is the best accepted one being the basis for uplifted analytical models. It benefits leaders, researchers, planners, etc. to build a guideline for forthcoming activities by reviewing the database to determine knowledge on current or past medical data proceedings [16]. This model does a detailed review of data to expose particulars like operation costs, cause for false steps and frequency of events. Descriptive analytics assists locating the root cause of the issue. Descriptive analytics also deal with it Proposed modal EEG classification [4].
The different analytical methods of data mining are
• Predictive Analysis
• Behavioral Analysis
• Data Interpretation.
1.1.3 Predictive Analysis
The probable questions in predictive analysis are
• In various domains, how does a data utilize the available data for predictive and real time analysis?
• How does a medical data make accuracy from unstructured data?
• How does a business influence unique varieties of data like social media data, sentiment data, multimedia, etc.?
1.1.4 Behavioral Analysis
Behavioral analysis deals with how a business influences complicated data to develop advanced models for
• Motivating results
• Making a medical budget
• Motivating revolution in medical approach
• Cultivating long-term consumer fulfilment.
1.1.5 Data Interpretation
The probable questions in data interpretation are
• What new analyses can be done from the available data?
• Which data should be analyzed for new product innovation?
Data classification is considered as a critical and challenging problem to be addressed in IoT medical data analytics. Classification is a method of labeling data for better productive usage depending upon necessity [34]. It functions with two paces: first includes learning activity and the second performs classification activity. The required data can be detected and obtained using well-organized classification model. The action of classifying the data using issues and difficulties opened by the data controllers is called data classification. Figure 1.2shows the paces connected to big data classification [30]. The different paces connected to classification are input data collection, data understanding, data shaping and data mining environment understanding. The success in data classification requires the understanding about design and structure of algorithms. It demonstrates activities such as configuration of huge data, management of big data and the methodology advancement related to classification [17]. The distinguishing parameters which impact the big data controller management and drive to issues in the advancement of learning layouts. Explores the paces associated with the machine learning algorithms and the flow of various phases is demonstrated. The cross validation and early stopping decision methods are applied for solving problems seen in the validation phase [16].
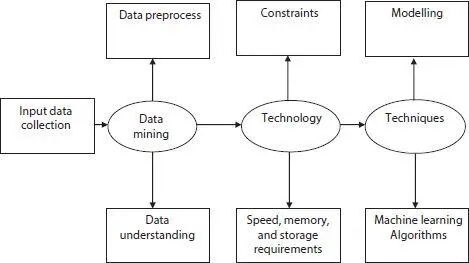
Figure 1.2 Data mining classification process.
Data classification is the task of applying computer vision and machine learning algorithms to extract meaning from a medical data. This could be as simple as assigning a label to the contents of an image, or data it could be as advanced as interpreting the contents of a data and returning a human-readable sentence [18]. Image and signal classification, at the very core, is the task of assigning a label to a data from a pre-defined set of categories. In practice, this means that given an input image, the task is to analyze the image and return a label that categorizes the image. This label is (almost always) from a pre-defined set. Open-ended classification problems are rarely seen when the list of labels is infinite [2].
In this proposed system examine about checking patient’s mind flags and recognizing the status of the patient progressively. To gather the information of cerebrum signals, we are utilizing Neurosky Mindwave Mobile Headset which deals with the EEG innovation. Figure 1.3demonstrate the proposed system design for EEG classification. It demonstrates the yield result in waveform design [33]. The overall system is given a multi-channel EEG stream in segments of 3 s every second, and a set of features are extracted at each time point and denoted as a sample. These samples are taken every second such that the subsequent window taken overlaps. As a result, the samples collected show a more gradual transition from one epileptic seizure state to the next [19]. A rectangular window is applied to each 3-second segment such that there is minimal distortion in the frequency response (some distortion will be present due to Gibb’s Phenomenon).
Читать дальше