After data pre-processing, normalization, feature extraction and feature reduction, different models are employed to predict the patient’s mortality in an in-hospital stage and calculate the accuracy. The models predict either patient will survive or die. This is determined by using classification technique as mortality prediction is a binary classification problem. This process is done step by step as shown in Figure 1.1.
Table 1.2 Time series variables with physical units [30].
S. no. |
Variables |
Physical units |
1. |
Temperature |
Celsius |
2. |
Heart Rate |
bpm |
3. |
Urine Output |
mL |
4. |
pH |
[0–14] |
5. |
Respiration Rate |
bpm |
6. |
GCS (Glassgow Coma Index) |
[3–15] |
7. |
FiO2 (Fractional Inspired Oxygen) |
[0–1] |
8. |
PaCo2 (Partial Pressure Carbon dioxide) |
mmHg |
9. |
MAP (Invasive Mean arterial blood pressure) |
mmHg |
10. |
SysABP (Invasive Systolic arterial blood pressure) |
mmHg |
11. |
DiasABP (Invasive Diastolic arterial blood pressure) |
mmHg |
12. |
NIMAP (Non-invasive mean arterial blood pressure) |
mmHg |
13. |
NIDiasABP (Non-invasive diastolic arterial blood pressure) |
mmHg |
14. |
Mechanical ventilation respiration |
[yes/no] |
15. |
NISysABP (Non-invasive systolic arterial blood pressure) |
mmHg |
1.3.5 Model Description and Development
Different models are developed in this chapter to estimate the performance of mortality prediction and comparison between them is also made. The models such as FLANN, Discriminant analysis, Decision Tree, KNN, Naive Bayesian and Support Vector Machine are applied to develop different classifiers. Out of 4,000 records of dataset A 3,000 records are taken as training set and remaining 1,000 records are used for validation or test of the models.
First of all Factor Analysis (FA) is applied to the selected variables to reduce the features. Factor analysis is one of the feature reduction techniques which is used to reduce the high dimension features to low dimension [31]. The 58 features of the dataset are reduced to 49 using FA. Several steps to of factor analysis are

Figure 1.1 Step by step process for mortality prediction.
1 First normalize the data matrix (Y) by using z-score method.
2 Calculate the auto correlation of the matrix (R).(1.1)
3 Calculate the Eigen vectors (U) and Eigen values (l)(1.2)
4 Rearrange the Eigen vector and Eigen values in descending order
5 Calculate the factor loading matrix (A) by using(1.3)
6 Calculate the score matrix (B)(1.4)
7 Calculate the factor score (F)(1.5)
After reducing the features, FLANN model [32] is used to predict patient’s survival or in-hospital death and finally evaluate the overall performance. The FLANN based mortality prediction model is shown in Figure 1.2. To design FLANN model 4,000 records of patients (dataset A) is selected. Out of 4,000 data, 3,000 data are selected for training and remaining 1,000 data are used for testing the model. During the training process each record with 49 features out of 3,000 records is taken as input. Each of the features is then expanded trigonometrically to five terms and map the data to a nonlinear format. The outputs of the functional expansion is multiplied with the corresponding weight valued and summed together to generate an output which is known as actual output. The actual output is then compared with the desired output either 0.1 (for 0) or 0.9 (for 1). If there are any differences in the actual and desired output, an error signal will be generated. On the basis of this error signal, weights and biases are updated using Least Mean Square (LMS) [33] algorithm. The process is repeated until all training patterns are used. The experiment is continued for 3,000 iterations. The value of learning parameter is 0.1. The mean square error (MSE) value for each iteration is stored and plotted to show the convergence characteristics as given in Figure 1.3. Once the training is over and the model is ready for prediction, 1,000 records which are kept aside for testing purpose in given to the model with fixed value of weights and bias obtained after the end of training process. For each input pattern the output or class label is calculated and compared with the target class label.
Figure 1.2 The FLANN based mortality prediction model.
Similarly, other models Discriminant Analysis (DA), Decision Tree (DT), K-Nearest Neighborhood (KNN), Naive Bayesian and Support Vector Machine (SVM) are also applied to predict mortality in an in-hospital death and obtained results using their own principles as briefed below.
Discriminant analysis [34] is one of the statistical tools which is used to classify individuals into a number of groups. To separate two groups, Discriminant Function Analysis (DFA) is used and to separate more than two groups Canonical Varieties Analysis (CVA) is used. There are two potential goals in a discriminant investigation: finding a prescient condition for grouping new people or deciphering the prescient condition to all the more likely comprehend the connections that may exist among the factors.
Decision Tree [35] is a tree like structure used for classification and regression. It is a supervised machine learning algorithm used in decision making. The objective of utilizing a DT is to make a preparation model that can use to foresee the class or estimation of the objective variable by taking in basic choice principles gathered from earlier data (training information). In DT, for anticipating a class name for a record one has to start from the foundation of the tree. We look at the estimations of the root property with the record’s characteristic. Based on correlation, one follows the branch and jump to the next node.
Figure 1.3 Convergence characteristics of FA-FLANN based mortality prediction model.
KNN [35] is also a supervised machine learning algorithm used for both classification and regression. It is simple and easy to implement algorithm. KNN finds the nearest neighbors by calculating the distance between the data points which is called the Euclidian distance.
A Naive Bayes classifier [35] is a probabilistic AI model that is utilized for classification task. The Bayes equation is given as
(1.6) 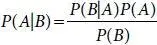
Utilizing Bayes hypothesis, it discovers the likelihood of an occurrence, given that B has happened. Here, B represents evidence and A represents hypothesis. The supposition made here is that the indicators/highlights are free. That is nearness of one specific element doesn’t influence the other. Consequently it is called Naïve.
Читать дальше