Handbook on Intelligent Healthcare Analytics
Здесь есть возможность читать онлайн «Handbook on Intelligent Healthcare Analytics» — ознакомительный отрывок электронной книги совершенно бесплатно, а после прочтения отрывка купить полную версию. В некоторых случаях можно слушать аудио, скачать через торрент в формате fb2 и присутствует краткое содержание. Жанр: unrecognised, на английском языке. Описание произведения, (предисловие) а так же отзывы посетителей доступны на портале библиотеки ЛибКат.
- Название:Handbook on Intelligent Healthcare Analytics
- Автор:
- Жанр:
- Год:неизвестен
- ISBN:нет данных
- Рейтинг книги:4 / 5. Голосов: 1
-
Избранное:Добавить в избранное
- Отзывы:
-
Ваша оценка:
- 80
- 1
- 2
- 3
- 4
- 5
Handbook on Intelligent Healthcare Analytics: краткое содержание, описание и аннотация
Предлагаем к чтению аннотацию, описание, краткое содержание или предисловие (зависит от того, что написал сам автор книги «Handbook on Intelligent Healthcare Analytics»). Если вы не нашли необходимую информацию о книге — напишите в комментариях, мы постараемся отыскать её.
The book explores the various recent tools and techniques used for deriving knowledge from healthcare data analytics for researchers and practitioners. A Handbook on Intelligent Healthcare Analytics
Handbook on Intelligent Healthcare Analytics — читать онлайн ознакомительный отрывок
Ниже представлен текст книги, разбитый по страницам. Система сохранения места последней прочитанной страницы, позволяет с удобством читать онлайн бесплатно книгу «Handbook on Intelligent Healthcare Analytics», без необходимости каждый раз заново искать на чём Вы остановились. Поставьте закладку, и сможете в любой момент перейти на страницу, на которой закончили чтение.
Интервал:
Закладка:
1
An Introduction to Knowledge Engineering and Data Analytics
D. Karthika* and K. Kalaiselvi†
Department of Computer Applications, Vels Institute of Science, Technology & Advanced Studies (Formerly Vels University), Chennai, Tamil Nadu, India
Abstract
In recent years, the philosophy of Knowledge Engineering has become important. Information engineering is an area of system engineering which meets unclear process demands by emphasizing the development of knowledge in a knowledge-based system and its representation. A broad architecture for knowledge engineering that manages the fragmented modeling and online learning of knowledge from numerous sources of information, non-linear incorporation of fragmented knowledge, and automatic demand-based knowledge navigation. The project aims to provide petabytes in the defined application domains with data and information tools. Knowledge-based engineering (KBE) frameworks are based on the working standards and core features with a special focus on their built-in programming language. This language is the key element of a KBE framework and promotes the development and re-use of the design skills necessary to model complex engineering goods. This facility allows for the automation of the process preparation step of multidisciplinary research (MDA), which is particularly important for this novel. The key types of design rules to be implemented in the implementation of the KBE are listed, and several examples illustrating the significant differences between the KBE and the traditional CAD approaches are presented. This chapter discusses KBE principles and how this technology will facilitate and enable the multidisciplinary optimization (MDO) of the design of complex products. This chapter discusses their reach goes beyond existing CAD structure constraints and other practical parametric and space exploration approaches. There is a discussion of the concept of KBE and its usage in architecture that supports the use of MDO. Finally, this chapter discusses on the key measures and latest trends in the development of KBE.
Keywords:Data analytics, knowledge, knowledge engineering, principles, knowledge acquisition
1.1 Introduction
1.1.1 Online Learning and Fragmented Learning Modeling
Applied artificial intelligence (AI) was defined as knowledge engineering [1], with three major scientific questions: knowledge representation, the use of information, and the acquisition of knowledge. In the big data age, the three fundamental problems must evolve with the basic characteristics of the complex and evolving connections between data objects, which are autonomous sources of information. Big data not only rely on domain awareness but also distribute knowledge from numerous information sources. To have knowledge of engineering tools for big data, we need tons of experience. Three primary research issues need to be addressed for the 54-month, RMB 45-million, 15-year Big Data Knowledge Engineering (BigKE) project sponsored by China’s Ministry of Science and Technology and several other domestic agencies: 1) online learning and fragmented learning modeling; 2) nonlinear fusion of fragmented information; and 3) multimedia fusion of knowledge. Discussing these topics is the main contribution of this article. With 1), we examine broken information and representation clusters, immersive online content learning with fragmented knowledge, and simulation with spatial and temporal characteristics of evolving knowledge. Question 2) will discuss connections, a modern pattern study, and dynamic integration between skills subsections of fragmented information. The key issues mentioned in Figure 1.1will be collaborative, context-based computing, information browsing, route discovery, and the enhancement of interactive knowledge adaptation.
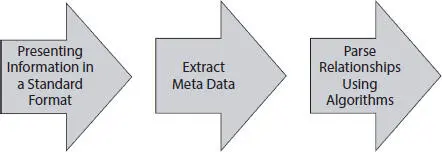
Figure 1.1 Knowledge engineering.
Due to these features of several channels, traditional offline data mining methods cannot stream data since it is important to reform the data. Online learning methods help solve this issue and adapt easily to the drift of the effects of streaming. But typical methods to online learning are explicitly configured for single-source info. Thus, the maintenance of these features concurrently provides great difficulties and opportunities for large-scale data production. Big data starts with global details, tackles dispersed data like data sources and function streams, and integrates diverse understanding from multiple data channels, as well as domain experience in personalized demand-driven knowledge services. In the age of big data, many data sources are usually heterogeneous and independent and require evolving, complex connections among data objects. These qualities are considered by substantial experience. Meanwhile, major suppliers of information provide personalized and in-house demand-driven offerings through the usage of large-scale information technologies [2].
Centered on the characteristics of multiple data sets, the key to a multisource retrieval of information is fragmented data processing [3]. To create global awareness, local information pieces from individual data points can be merged. Present online learning algorithms often use linear fitting for the retrieval of dispersed knowledge from local data sources [4]. In the case of fragmented knowledge fusion, though, linear fitting is not successful and may even create problems of overfitting. Several studies are ongoing to improve coherence in the processing and interpretation of fragmented knowledge [6], and the advantage of machine learning for large data interpreting is that most samples are efficient, thus eliminating the possibility of over-adjustment at any rate [7]. Big data innovation acquires knowledge mostly from user-produced content, as opposed to traditional information engineering’s focused on domain experience, in addition to authoritative sources of knowledge, such as technical knowledge bases. The content created by users provides a new type of database that could be used as a main human information provider as well as to help solve the problem of bottlenecks in traditional knowledge engineering. The information created by the consumer is broad and heterogeneous which leads to storage and indexing complexities [5], and the knowledge base should be able to build and disseminate itself to establish realistic models of data relations. For instance, for a range of reasons, clinical findings in survey samples can be incomplete and unreliable, and preprocessing is needed to improve analytical data [8].
Both skills are essential for the creation of personalized knowledge base tools as the knowledge base should be targeted to the needs of individual users. Huge information reinforces distributed expertise to develop any ability. Big data architecture also requires a customer interface to overcome user-specific problems. With the advent of science and innovations, in the fast-changing knowledge world of today, the nature of global economic growth has changed by introducing more communication models, shorter product life cycles, and a modern product production rate. Knowledge engineering is an AI field that implements systems based on information. Such structures provide computer applications with a broad variety of knowledge, policy, and reasoning mechanisms that provide answers to real-world issues. Difficulties dominated the early years of computer technology. Also, knowledge engineers find that it is a very long and expensive undertaking to obtain appropriate quality knowledge to construct a reliable and usable system. The construction of an expert system was identified as a knowledge learning bottleneck. This helped to gain skills and has been a big area of research in the field of information technology.
Читать дальшеИнтервал:
Закладка:
Похожие книги на «Handbook on Intelligent Healthcare Analytics»
Представляем Вашему вниманию похожие книги на «Handbook on Intelligent Healthcare Analytics» списком для выбора. Мы отобрали схожую по названию и смыслу литературу в надежде предоставить читателям больше вариантов отыскать новые, интересные, ещё непрочитанные произведения.
Обсуждение, отзывы о книге «Handbook on Intelligent Healthcare Analytics» и просто собственные мнения читателей. Оставьте ваши комментарии, напишите, что Вы думаете о произведении, его смысле или главных героях. Укажите что конкретно понравилось, а что нет, и почему Вы так считаете.